Marlin Ulmer
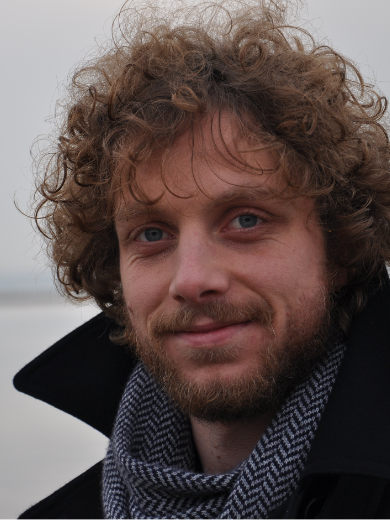
Prof. Dr. Marlin Ulmer
Session: Supply Chain & Sustainability Management
Title: Optimization for Stochastic
Dynamic Decision Processes
Marlin Ulmer is professor for Management Science at the Otto-von-Guericke-Universität Magdeburg. He studied Mathematics at the University of Göttingen and received his PhD in Business Administration from the Technische Universität Braunschweig. Before moving to Magdeburg, Marlin was a Junior-Professor at TU Braunschweig and a DFG Emmy Noether Fellow at TU München. He also spend one semester each at the University of Iowa and at Georgia Tech. Marlin Ulmer’s primary research interests are decision support for mobility and logistics, stochastic dynamic decision problems, data-driven optimization, and Machine Learning. Particular applications are the tactical planning and operational control of service fleets under various sources of uncertainty and with several stakeholders, shared mobility, services with consistency considerations, and sustainable last mile city logistics. He works on the development and design of computational methods of Operations Research, Machine Learning, as well as their combinations. Marlin Ulmer’s work is well-recognized in the international research community and received a variety of national and international awards.
Optimization for Stochastic Dynamic Decision Processes
In modern supply chain and logistics operations, planning is often done under incomplete information and plans are subject to updates once more information is revealed. For example, new demand may come in while operations are already in process, parts of the operations are unexpectedly delayed, or resource availability may change. All this requires dynamic updates of previous planning and changes the nature of decision making. Instead of finding the most efficient plans (already a quite complex endeavor), flexibility and anticipation of future changes become essential for effective operations. In this talk, we will introduce such stochastic dynamic decision processes and give an overview of the main solution strategies, amongst others, simulation approaches and reinforcement learning. With a few examples from ongoing research projects, we will illustrate that there is no “one size fits all”-approach, but that data and problem structure play an important role.